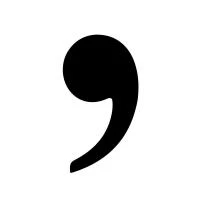
path
stringlengths 38
40
|
---|
3b41c0fa8959aea6c118e5714f412a2e_13.npy |
514520784bd7b5e0cfacddce40092428_7.npy |
aa4887f49431bcb803c00b93acc3a018_10.npy |
db69bc3bf1827a76b44cbf6114bf1581_31.npy |
2c227b3b141bd45319c5a48d020954f2_13.npy |
774403dc5aeaf1323fc671832735a1e3_214.npy |
57de94288a86a998b7e45ec5c991965e_1.npy |
0e7f939462b833a5cd485aa9ff8fea8f_16.npy |
1bf30722776c8c9b4c389f7b60503122_8.npy |
206461e1ace4da5140da1e1bbd377297_24.npy |
2fd3114472f69b87f6473b52046d4b4f_27.npy |
b660c0dc3c04c17cbba56d253d7a9fc9_4.npy |
f0bdd51d73e6e35b3f30d2a39e7de5f2_10.npy |
82f245f8a61f344dee69ec669869a2c9_17.npy |
306a843bf0d5e8432076f88631fcb871_6.npy |
fa7ee9eb8d48e55b9db9bb763a969013_10.npy |
3443b96d21ac877daa46c34bbddaa09b_6.npy |
d08232e8180eb11dc7f80e7be2d4cd4a_2.npy |
69cd1dfa915bdbb5f13f32e72a1e4ca9_21.npy |
131aa5482de6d37cb301b2cfb210dda8_19.npy |
5ddd4a8603dc25c5a47b7a72972edb3c_12.npy |
966fdf10c88098369920248904116d7a_169.npy |
b0b0a7a72f8ab63d3aead0980f5d5cf5_6.npy |
3fbec25841065d6ea8f2973483c2e860_3.npy |
6b76985d8d6da88416b133361c681f85_63.npy |
8a821fc82edbcbcf2c7892a2e09ad34c_25.npy |
7bc943af724324316024dac17ada24d1_64.npy |
60413816432f5ebdeaccfeeac151dfcb_2.npy |
e999b87a78ced279bdf887edafdb5f5d_6.npy |
37e892c5c27e8dbc239ab307619a8a9a_3.npy |
b8f746af75cbd481812e43260ce69e46_5.npy |
a90f35237e9b6ebb903f012ed3cb94c5_76.npy |
7664ac276248e10bbbad6b46873cc86b_63.npy |
23617ea465e8c4a014a360fe003c9688_5.npy |
6586ec95e99d635a5e7091aed3c728d4_0.npy |
9f63b8679654ff002ee411cd5e7f3a0d_23.npy |
038c874f2a5c51f36171e0d504cc04cc_13.npy |
cf11ad7815a49d438cecbc0ba27ff31b_48.npy |
d2c06243589bf9b98a54341279b081bb_6.npy |
b98b520656473dabad0f1b9b624855ff_11.npy |
e2c09cdb57045857bcfbb8836ca58b67_14.npy |
6baa4e5af260504078d0005e84884b45_8.npy |
dc0f4795808e22089b36f92ccee06e91_6.npy |
7f2572917e6f29bcecaa96acd20a98c4_6.npy |
b2d977173d0c502f0e19ce8c9660d42b_6.npy |
50722362feea7a344aa17bbac70212bb_4.npy |
6f71500cc2686174f45312dd72ee5272_45.npy |
9f0fe4e90a35a0773a80e4a6c1c80d89_20.npy |
3f1402d02a298c00b2065b326afc30e9_3.npy |
7d616267c4aacff0f41feb1065f0df1a_83.npy |
ffb1035fe1454ab3ebfccad8124c327f_15.npy |
c406c512c66e4ac21b548b6f958cce5a_6.npy |
34973a46a6c0bcd1d576c3c680802953_12.npy |
ccc0d965a41af37a1bff9a3788dac4c3_26.npy |
023ae07fda627a30d0f10ac86735692a_65.npy |
4a090106631f20de92365b293ccbc5f4_13.npy |
8fd2816d2d925d0d6b6ffadbd6602147_1.npy |
6796d15e53b11a231e451cd97fc0e8dc_59.npy |
8230d06e4b6ab5ea66ac34ca21209a4c_53.npy |
289cc7b78e9a2dff8a331db293c8ddfd_43.npy |
54e914bf7fdf798fbbe6708cd6a0498a_5.npy |
d5f85000374bc0ecfb35e5b854f44e65_5.npy |
a53ca51260369096abac08b3721e30bd_0.npy |
0ad8fd36693721f2794959688b2f51c9_55.npy |
3ad1cf1676796a8edadef70810422129_3.npy |
bd631298a58d89665f13177be4dc67ed_7.npy |
88fee5ab2b15ed8f460fb91012fc2044_19.npy |
a99c908a3cdcbb91bc1eaa3d889c0ef8_29.npy |
1c1a8567818977632e1c903766db3b1a_7.npy |
c140741008cffa59309ee0924a819571_0.npy |
c1599c49f5ba277664fd66962c436bac_42.npy |
a4ad97e9cabe78f4941973abcddc0778_4.npy |
48966d3272fbc8c9a8bd8ee21a83d321_14.npy |
10840117907bc6b825b47bed360e4619_22.npy |
b4ef5ce20fe2df0fee14f354acd53d27_24.npy |
04feb3a5e341965207fb84e2a8d73657_41.npy |
1ff78a5226d679869ebe372ca979e2ab_7.npy |
3239d15c2c28c2396611f7721e3d3280_30.npy |
d6ea0fe460fd91a01868f5928f45a682_2.npy |
c12b9367b20e4b559f26749927767d0e_2.npy |
f4efa917a2a2e9d0c5e4bc12a31ec37b_10.npy |
987d025555b4fd7c5902654297982242_3.npy |
38df9fdc8f316fd89c799a432ef3434e_6.npy |
bba892d5ba82269fc247606ee1df33ff_2.npy |
239b80dd6d4423baaf1d56ad8dcb87c2_4.npy |
6541f2fde1ec35effefa030d62a85b9c_11.npy |
cfc0b9b6e49b263618f73187c88ccdf6_3.npy |
d4c0558467604eb9da826343785014d4_22.npy |
df48107ddba8a08bf5ecd98bbd964734_7.npy |
64f83f6d95303d2bb449ea8d9e5301cb_94.npy |
044646730f2e07d9c65ad5f0510573ce_7.npy |
3dac1d280aff8837d422d4aab2ca42df_3.npy |
613afd8691a4537122af5a6eda8b726a_8.npy |
a6af26234b8f1cd22354ab06ab1d9de7_40.npy |
dffb9dfe98b378b61db8506e04cdd6d9_68.npy |
3a91c9874707e5a664c85c5d9bed46e9_10.npy |
b8cd65f45a10e6c4f6b341fc79a322e1_2.npy |
cf762dbf79ac69598a7c771590405eae_8.npy |
b44ddb9dddaa36a5c00588679d46a7e7_21.npy |
55c41db0b7e41ecd4a7aa3a39abdc873_3.npy |
commaVQ
commaVQ is a dataset of 100,000 heavily compressed driving videos for Machine Learning research. A heavily compressed driving video like this is useful to experiment with GPT-like video prediction models. This repo includes an encoder/decoder and an example of a video prediction model. Examples and trained models can be found here: https://github.com/commaai/commavq
Overview
A VQ-VAE [1,2] was used to heavily compress each frame into 128 "tokens" of 10 bits each. Each entry of the dataset is a "segment" of compressed driving video, i.e. 1min of frames at 20 FPS. Each file is of shape 1200x8x16 and saved as int16.
Note that the compressor is extremely lossy on purpose. It makes the dataset smaller and easy to play with (train GPT with large context size, fast autoregressive generation, etc.). We might extend the dataset to a less lossy version when we see fit.
References
[1] Van Den Oord, Aaron, and Oriol Vinyals. "Neural discrete representation learning." Advances in neural information processing systems 30 (2017).
[2] Esser, Patrick, Robin Rombach, and Bjorn Ommer. "Taming transformers for high-resolution image synthesis." Proceedings of the IEEE/CVF conference on computer vision and pattern recognition. 2021.
- Downloads last month
- 1,525
Models trained or fine-tuned on commaai/commavq
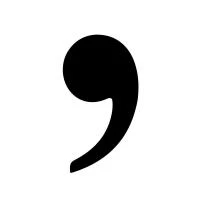